
- This event has passed.
Uncovering personalized glucose responses and circadian rhythms from multiple wearable biosensors with Bayesian dynamical modeling
August 7, 2024 @ 4:00 pm - 5:00 pm KST
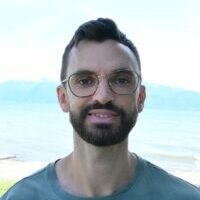
Abstract:
Wearable biosensors measure physiological variables with high temporal resolution over multiple days and are increasingly employed in clinical settings, such as continuous glucose monitoring in diabetes care. Such datasets bring new opportunities and challenges, and patients, clinicians, and researchers are today faced with a common challenge: how to best summarize and capture relevant information from multimodal wearable time series? Here, we aim to provide insights into individual glucose dynamics and their relationships with food and drink ingestion, time of day, and coupling with other physiological states such as physical and heart activity. To this end, we generate and analyze multiple wearable device data through the lens of a parsimonious mathematical model with interpretable components and parameters. A key innovation of our method is that the models are learned on a personalized level for each participant within a Bayesian framework, which enables the characterization of interindividual heterogeneity in features such as the glucose response time following meals or underlying circadian baseline rhythm. I will also describe how we are currently applying this framework in the context of gestational diabetes.