
- This event has passed.
Quantifying dynamical changes in sparse, noisy, high-dimensional data
November 18, 2022 @ 11:00 am - 12:00 pm KST
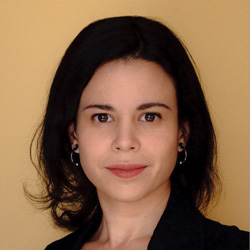
The circadian clock orchestrates a vast array of behavioral and physiological processes with a 24-hour cycle, enabling nearly all organisms — from bread mold to fruit-flies to humans — to anticipate and adapt to the Earth’s day. Entrainable by environmental cue, the rhythm itself is generated by a self-sustained molecular oscillator present in nearly every cell. This in turn governs the expression of thousands of genes, precisely coordinating biomolecular functions at the microscopic scale. While experimental evidence suggests that the clock is crucial for mediating the response to changes in an organism’s environment (such as temperature and food availability), the precise mechanisms underlying circadian regulation remain unclear. Today, high-throughput omics assays enable us to probe these processes in molecular detail, with the goal of making inferences about which genes are under circadian control and how their dynamics change under different environmental conditions. Analyzing this transcriptomic time-series data raises new challenges: that of characterizing dynamics when the data are noisy, sparsely sampled in time, and may not be strictly periodic. In this talk, I will discuss our recent work on nonparametric methods to analyze circadian transcriptomic data by exploiting results from dynamical systems theory, nonlinear dimension reduction, and topological data analysis.