- AlphaFold2 predicts structural effects of mutations that are correlated with changes in stability
-
Researchers at the Center for Algorithmic and Robotized Synthesis within the Institute for Basic Science have
taken a significant step forward in understanding the stability of proteins by leveraging the power of AI. The
research team used AlphaFold2 to explore how mutations affect protein stability—a crucial factor in ensuring
proteins function correctly and do not cause diseases like Alzheimer's.
DeepMind’s AlphaFold algorithm, which can accurately predict a protein’s structure from its gene, has been a
game-changer across the field of biology, making structural biology accessible to everyone. Despite this
immense success, two fundamental questions remain unanswered: Will the predicted structures fold correctly and
stay folded? And a general question about AI algorithms: how does AlphaFold actually work?
A critical limitation of AlphaFold is that it was trained on a set of stable proteins that stay folded at
physiological temperatures. As a result, it predicts the most likely folded structure without knowing if it
will certainly fold or will be unstable. Knowing and predicting protein stability is crucial because unstable
proteins can misfold, leading to dysfunction and potentially serious diseases, so the cells must spend much
energy to get rid of them. Furthermore, most proteins are only marginally stable, making them highly
susceptible to mutations that can cause them to unfold. Thus, protein engineering is much about careful
navigation in a minefield of dysfunctional protein sequences that do not fold. All this implies that the next
step in using AlphaFold should be to try to predict those changes in stability due to mutations.
A fundamental question tested in this study was whether AlphaFold has learned the underlying physics of
protein folding or is simply a high-dimensional regression machine that merely recognizes statistical
patterns. This question is about the capacity to generalize: if AlphaFold has somehow learned the physical
forces in action, it ought to work on protein sequences it has not seen before.
That’s exactly what the two IBS researchers, John MCBRIDE and Tsvi TLUSTY, wanted to test in their study.
Their way to tackle this question was to examine if AlphaFold can correctly predict the effects of mutations
on stability. There are infinitely more mutations than data points used in the training of AlphaFold, meaning
that even very sophisticated regression will not suffice to account for the full range of mutation effects.
This task is highly challenging since critical changes in stability often involve small structural changes
that are hard to predict. Still, it turns out that there are some useful clues within the structural changes
predicted by AlphaFold that provide valuable information on possible stability changes.
IBS researchers showed this by comparing structural changes inflicted by mutations to differences in
experimentally measured stability between the wild-type and mutated protein [1]. A critical ingredient was
using a probe that is very sensitive to small changes. The researchers devised an innovative metric, known as
the effective strain, [2] to detect small but important changes in protein structure that are linked to
stability.
Looking at thousands of mutations, they found that the effective strain measure correlates with the magnitude
of the change in stability. That is to say, large structural changes (predicted by AlphaFold) also predict
large changes in stability.
Lead author John MCBRIDE stated, “This is a strong indication that the structures predicted by AlphaFold
encode significant physical information, particularly about stability. It is necessary to develop new physical
models to decode this information further.”
These insights open up new possibilities for protein engineering, a field that involves designing proteins
with specific functions. By better understanding how mutations affect stability, scientists can navigate the
complex landscape of protein design more effectively, potentially leading to advances in drug development and
treatments for diseases caused by protein misfolding.
This research marks an important milestone in the ongoing exploration of how AI can be used to unravel the
complexities of biology and underscores the need for further studies to fully unlock the potential of AI in
scientific discovery.
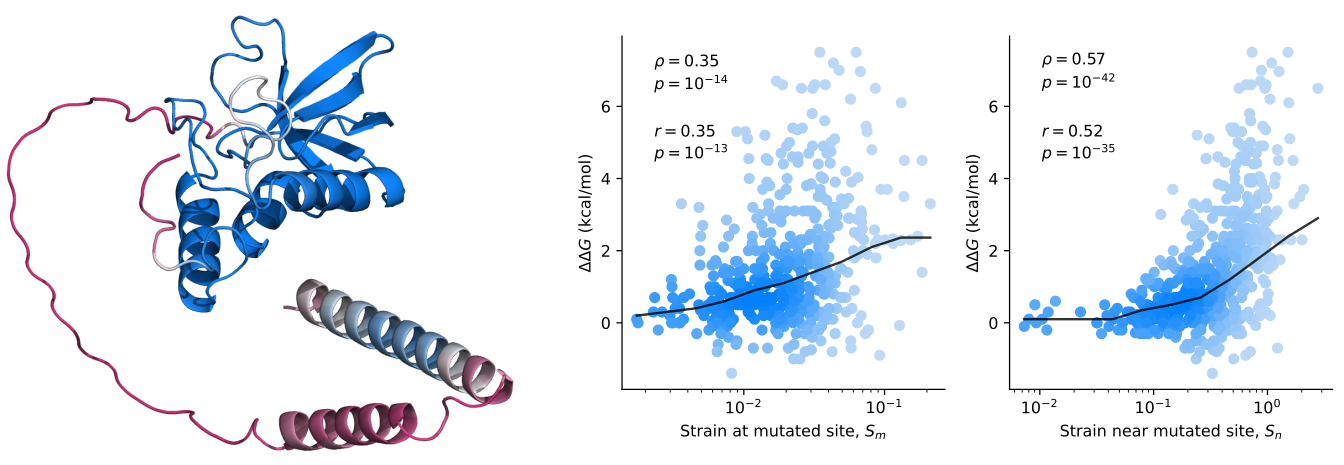
Figure
1. A: Structure of Thermonuclease from Staphylococcus aureus predicted by AlphaFold2. Change in stability upon
mutation, ∆∆G (positive values are destabilizing), is plotted as a function of effective strain measured at
the mutated site, and in a spherical region near the mutated site, for 491 mutants. The black line shows the
median. Statistical correlations are shown for Pearson’s r and Spearman’s ρ.
Notes for editors
- References
[1] McBride and Tlusty, AI-predicted protein deformation encodes energy landscape, PRL 2024
[2] McBride et al., AlphaFold2 can predict single-mutation effects, PRL 2023
- Media Contact
For further information or to request media assistance, please contact William I. Suh at the IBS Public
Relations Team (willisuh@ibs.re.kr).
- About the Institute for Basic Science (IBS)
IBS was founded in 2011 by the government of the Republic of Korea with the sole purpose of driving
forward the development of basic science in South Korea. IBS has 7 research institutes and 31 research
centers as of June 2024. There are eight physics, three mathematics, five chemistry, seven life science, two
earth science, and six interdisciplinary research centers.